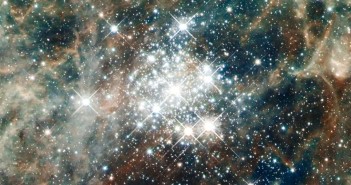
Catching Galactic Recycling In The Act
Editor’s note: Astrobites is a graduate-student-run organization that digests astrophysical literature for undergraduate students. As part of the new partnership between the AAS and astrobites, we will be reposting astrobites content here at AAS Nova once a week. We hope you enjoy this post from astrobites; the original can be viewed at astrobites.org!
Title: ALMA Reveals Potential Localized Dust Enrichment from Massive Star Clusters in II Zw 40
Authors: S. Michelle Consiglio, Jean L. Turner, Sara Beck, David S. Meier
First Author’s Institution: University of California, Los Angeles
Status: Published in ApJL, open access
Galaxies are recycling centers for gas. Dense gas collapses under gravity to form stars. When massive stars form, they quickly impact their surroundings with intense radiation and mass loss. This enriches the galaxy with heavy elements forged inside the massive stars and becomes the raw material for future star and planet formation. The evolution of the galaxy is determined by this cycle of gas to stars and back.
To measure the lifecycle of gas in galaxies, astronomers use radio telescopes that are sensitive to the three main phases of gas. First, the dense gas where stars form emits light with millimeter-size wavelengths from carbon monoxide (CO). Next, gas ionized by massive stars gives off radio waves when particles collide, in a process called free-free emission. Finally, heavy elements lost by massive stars, which will fuel the next generation of star formation, coalesce to form grains of dust that emit light in the submillimeter regime. With an unmatched angular resolution, the Atacama Large Millimeter/submillimeter Array (ALMA) is the tool of choice for studying the cycle of gas in galaxies.
The authors of today’s paper used ALMA to measure the gas and dust in the nearby galaxy II Zw 40. This small galaxy is forming stars at a prodigious rate. The starlight in the galaxy is dominated by massive stars. The authors investigate the effect of these stars on the gas and dust in the galaxy.
Observations of Gas and Dust
With ALMA, the authors observed three different components of II Zw 40. At a wavelength of 3 mm, the dominant source is free-free emission from ionized gas around the massive star cluster. At 870 µm, after accounting for free-free emission, most of the light is emitted by dust grains. These two components are shown in Figure 1. The peaks of ionized and dust emission are distinct, and the dust emission is localized in several clumps around the cluster.
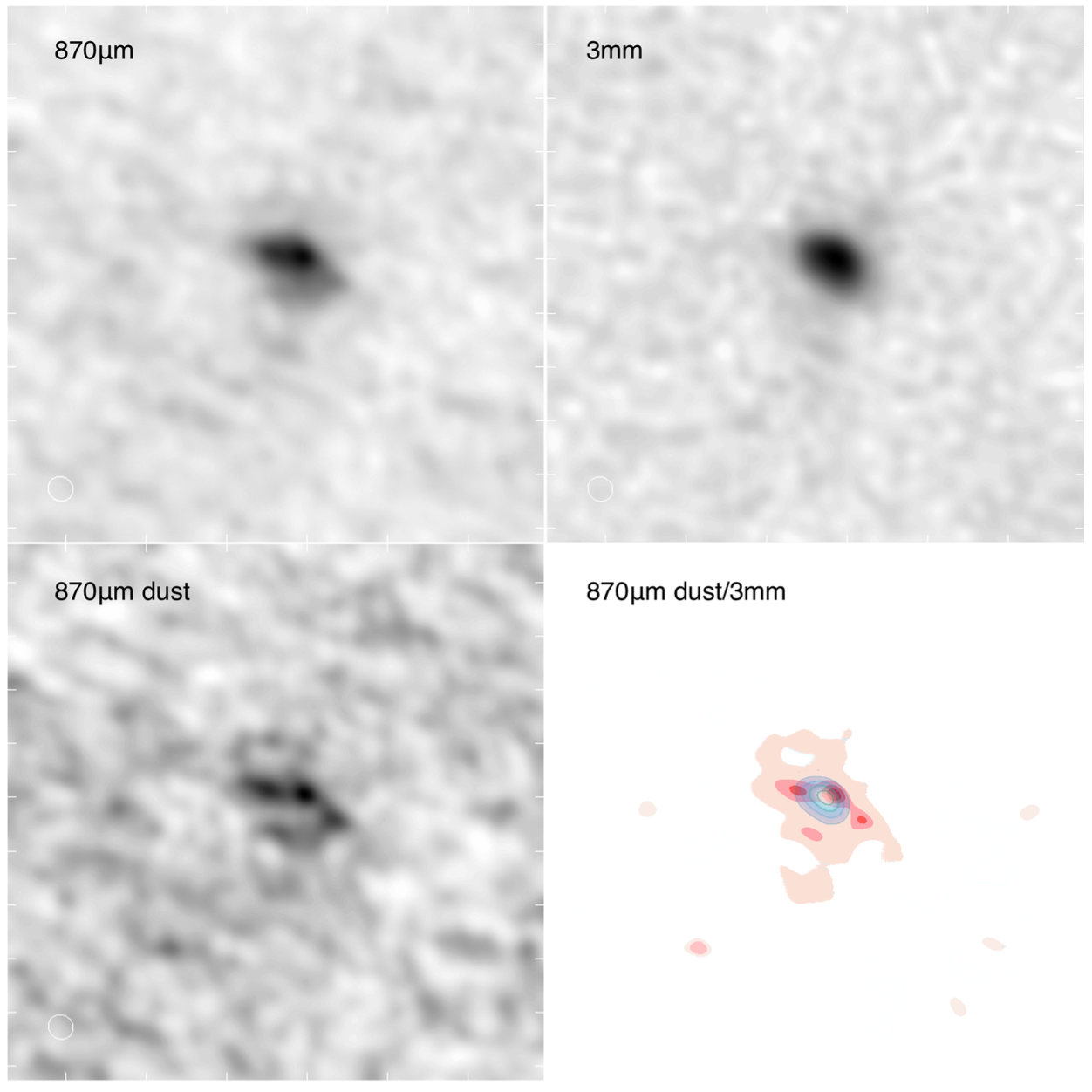
Figure 1: The galaxy as seen at 3 mm and 870 µm wavelengths. The 3 mm map (upper right and blue contours) shows the extent of free-free emission from ionized gas around massive stars. Removing the free-free contribution from the total 870 µm emission (upper left) gives a map of dust emission (lower left and red contours). [Consiglio et al. 2016]
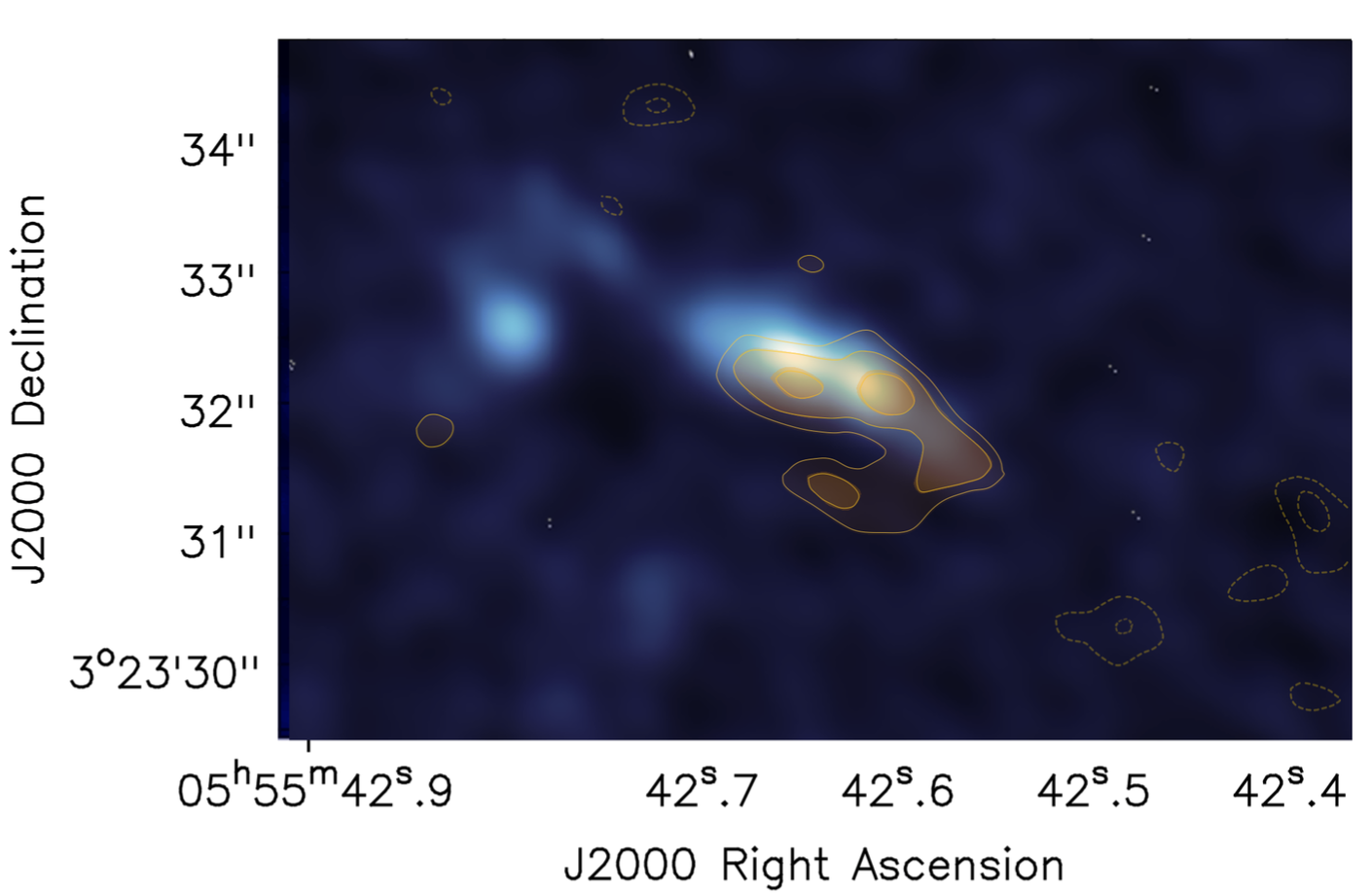
Figure 2: Dense gas and dust emission in the galaxy. Dense gas is traced by emission from CO molecules (blue map). Dust emission is traced by 870 µm emission (orange contours, same as lower left panel in Figure 1). Note the discrepancy between the peaks of dense gas and dust. [Consiglio et al. 2016]
Clumps of Stardust
To explain the low, variable gas-to-dust ratio and the clumpy structure of the dust emission, the authors propose that massive stars enrich nearby clouds with heavy elements and dust. Because this enrichment is ongoing, more dust is joining the dense gas, lowering the total gas-to-dust ratio. The dust is clumpy because it has not yet mixed with the rest of the galaxy. This enrichment model suggests that dust without associated dense gas came from an older star cluster that is not visible in free-free emission (Figure 1) because it no longer ionizes gas.
Pushing Dust With Light
Take another look at Figure 1, and notice how the peaks of dust emission are all slightly offset from the peak of the ionized gas. In addition to ionizing gas, the intense radiation from massive stars can actually push on dust grains. Acting like a multitude of tiny billiard balls, the photons from bright stars exert radiation pressure on the dust. The dust then drifts relative to the gas, which may explain the offset between gas and dust peaks.
ALMA has revolutionized the study of star formation in galaxies. This paper shows that the cycle from dense gas (traced by CO) to massive stars (3 mm) to dust-rich gas (870 µm) is complex. The massive stars in the galaxy have enriched parts of the galaxy, while other areas remain relatively dust-free. The dust-rich products of stellar evolution are pushed by radiation pressure but have not yet mixed into the galaxy. Future studies of the galactic recycling plant will explain the origin and dispersal of the ingredients needed for planets and (perhaps) life. Cue Carl Sagan.
About the author, Jesse Feddersen:
I am a 4th-year graduate student at Yale, where I work with Héctor Arce on the effect of stellar feedback on nearby molecular clouds. I’m a proud Indiana native (ask me what a Hoosier is), and received my B.S. in astrophysics from Indiana University. If I’m not working, you’ll probably find me on a trail or at a concert somewhere.