One of the major challenges for modern supernova surveys is identifying the galaxy that hosted each explosion. Is there an accurate and efficient way to do this that avoids investing significant human resources?
Why Identify Hosts?
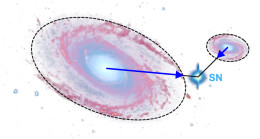
One problem in host galaxy identification. Here, the supernova lies between two galaxies — but though the centroid of the galaxy on the right is closer in angular separation, this may be a distant background galaxy that is not actually near the supernova. [Gupta et al. 2016]
But how do we identify which galaxy hosted a supernova? This seems like a simple problem, but there are many complicating factors — a seemingly nearby galaxy could be a distant background galaxy, for instance, or a supernova’s host could be too faint to spot.
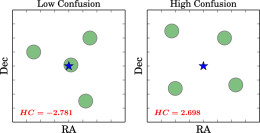
The authors’ algorithm takes into account “confusion”, a measure of how likely the supernova is to be mismatched. In these illustrations of low (left) and high (right) confusion, the supernova is represented by a blue star, and the green circles represent possible host galaxies. [Gupta et al. 2016]
Turning to Automation
Before the era of large supernovae surveys, searching for host galaxies was done primarily by visual inspection. But current projects like the Dark Energy Survey’s Supernova Program is finding supernovae by the thousands, and the upcoming Large Synoptic Survey Telescope will likely discover hundreds of thousands. Visual inspection will not be possible in the face of this volume of data — so an accurate and efficient automated method is clearly needed!
To this end, a team of scientists led by Ravi Gupta (Argonne National Laboratory) has recently developed a new automated algorithm for matching supernovae to their host galaxies. Their work builds on currently existing algorithms and makes use of information about the nearby galaxies, accounts for the uncertainty of the match, and even includes a machine learning component to improve the matching accuracy.
Gupta and collaborators test their matching algorithm on catalogs of galaxies and simulated supernova events to quantify how well the algorithm is able to accurately recover the true hosts.
Successful Matching
![The matching algorithm’s accuracy (“purity”) as a function of the true supernova-host separation, the supernova redshift, the true host’s brightness, and the true host’s size. [Gupta et al. 2016]](https://aasnova.org/wp-content/uploads/2016/11/fig44-260x161.jpg)
The matching algorithm’s accuracy (“purity”) as a function of the true supernova-host separation, the supernova redshift, the true host’s brightness, and the true host’s size. [Gupta et al. 2016]
The encouraging results of this work — which was intended as a proof of concept — suggest that methods similar to this could prove very practical for tackling future survey data. And the method explored here has use beyond matching just supernovae to their host galaxies: it could also be applied to other extragalactic transients, such as gamma-ray bursts, tidal disruption events, or electromagnetic counterparts to gravitational-wave detections.
Citation
Ravi R. Gupta et al 2016 AJ 152 154. doi:10.3847/0004-6256/152/6/154
1 Comment
Pingback: matching a SN to the host galaxy is not trivial