In 2006 an ambitious project was begun: creating the world’s largest telescope with the goal of imaging the shadow of a black hole. But how will we analyze the images this project produces?
A Planet-Sized Telescope
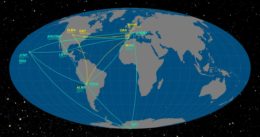
The locations of the participating telescopes of the Event Horizon Telescope (EHT) and the Global mm-VLBI Array (GMVA) as of March 2017. Jointly, these telescopes plan to image the shadow of the event horizon of the supermassive black hole at the center of the Milky Way. [ESO/O. Furtak]
The EHT, researchers hope, will have the power to peer in millimeter emission down to the very horizon of an accreting black hole — specifically, Sgr A*, the supermassive black hole in the Milky Way’s center — to learn about black-hole physics and general relativity in the depths of this monster’s gravitational pull.
Today, the EHT is closer than ever to its goal, as the project continues to increase its resolving power and sensitivity as more telescopes join the system. Another important aspect of this project exists, however: the ability to analyze and characterize the images it produces in a meaningful way.
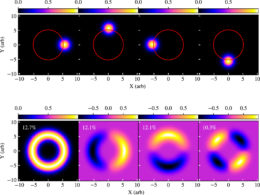
A simple example of using principal component analysis to decompose a set of images into independent eigenimages. The example images (top row) are snapshots from a simple model of a Gaussian spot moving on a circular path. The first four components of the principal component analysis decomposition — the four leading eigenimages — are shown in the bottom row, labeled with their corresponding eigenvalues. [Adapted from Medeiros et al. 2018]
Principal Components
Principal component analysis is a clever mathematical approach that allows the user to convert a complicated set of observations of variables into their “principal components”. This process — commonly used in traditional statistical applications like economics and finance — can simplify the amount of information present in the observations and help identify variability.
Medeiros and collaborators demonstrate that a time sequence of simulated EHT observations — produced from high-fidelity general-relativistic magnetohydrodynamic simulations of a black hole — can be decomposed using principal component analysis into a sum of independent “eigenimages”. These eigenimages provide a means of compressing the information in the snapshots: most snapshots can be reproduced by summing just a few dozen of the leading eigenimages.
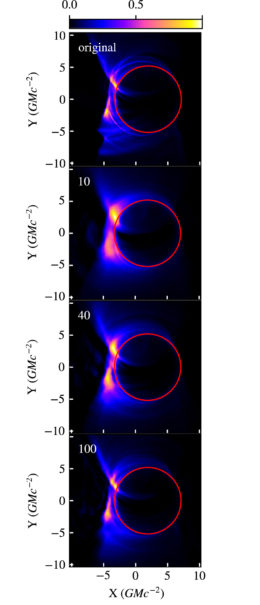
A typical snapshot from a simulation (top), followed by three different reconstructions of the snapshot from the leading 10, 40, and 100 eigenimages. [Adapted from Medeiros et al. 2018]
Exploring Steady and Variable Flow
How is this useful? If images from simulations of a black hole can be represented by sums of eigenimages, so can the actual observations produced by the EHT. By comparing the two sets of observations — real and simulated — to each other within this eigenimage framework, we’ll be able to better understand the components of what we’re observing. In addition, the mathematics of principal component analysis allow for this to work even with sparse interferometric data, as is expected with EHT observations.
Furthermore, recognizing images that aren’t represented well by the leading eigenimages is equally important. These outlier images can be indicative of flaring or otherwise variable phenomena around the black hole, and identifying moments in which this occurs will help us to better understand the physics of accretion flows around black holes.
So keep an eye out for the first images from the EHT, expected soon — there’s a good chance that principal component analysis will be helping us to make sense of them!
Citation
“Principal Component Analysis as a Tool for Characterizing Black Hole Images and Variability,” Lia Medeiros et al 2018 ApJ 864 7. doi:10.3847/1538-4357/aad37a
3 Comments
Pingback: AAS Nova – New
Pingback: | BH PIRE
Pingback: Research Highlight | Feryal Özel