Editor’s Note: Astrobites is a graduate-student-run organization that digests astrophysical literature for undergraduate students. As part of the partnership between the AAS and astrobites, we occasionally repost astrobites content here at AAS Nova. We hope you enjoy this post from astrobites; the original can be viewed at astrobites.org.
Title: Disentangling CO Chemistry in a Protoplanetary Disk Using Explanatory Machine Learning Techniques
Authors: Amina Diop et al.
First Author’s Institution: University of Virginia
Status: Published in ApJ
As the birthplaces of planets, protoplanetary disks hold many useful clues for how planets form and evolve. One of the pieces of information we can gather from disks is composition — to date, more than 35 different molecules have been detected in disks (see this bite for a summary of how these molecules are detected). Ideally, we want to be able to connect the abundances of different molecules to disk properties, such as total mass. This is more easily said than done, however, as a lot of different factors can alter the observed abundances of different molecular species. For example, one of the molecules we arguably have the best chemical understanding of is CO (carbon monoxide), which has been studied in a number of protoplanetary disks. Nonetheless, measured CO abundances tend to be lower than expected, indicating that effects such as local variations in temperature and dust distributions throughout the disk may have a significant impact on the observed abundances.
In order to try to understand the connection between local disk environments and molecular abundances, studies typically simulate a disk many times over, running through a huge range of different disk properties. As you might imagine, this approach is slow and takes a lot of computational resources. Today’s authors are trying a new method, using machine learning to more quickly find connections between disk properties and abundances. The goal is to use machine learning to search large parameter spaces and understand which physical disk properties are most important to creating and destroying CO in disks.
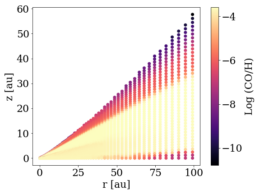
Figure 1: The initial CO abundances relative to hydrogen throughout the disk. You can think of r and z as x and y coordinates in the disk, where the star would be at (0,0). [Diop et al. 2024]
Making a Disk Model
The authors start with a disk around a T Tauri star (a type of young variable star that could be a typical host to disks). They assign a corresponding temperature and X-ray luminosity to the star, and they assume the disk is a mix of gas and dust, with both large and small dust grains. After assuming some initial compositions for the disk, they then apply a complex chemical code, including several thousand (!) different reactions to the modeled disk to calculate the CO abundances over 3 million years. Figure 1 shows the initial distribution of CO in one of these models.
Bring on the Machine Learning!
The authors consider each CO abundance calculated in the disk as a separate sample (so each point in Figure 1 is a sample) and check how each point’s CO value compares to local disk properties at that point, such as temperature, ultraviolet flux, and gas density. Figure 2 shows one example, with CO abundances plotted for specific temperatures and gas densities throughout the disk.
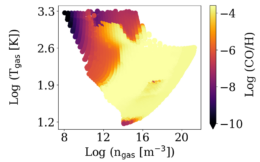
Figure 2: The disk from Figure 1, a million years later. The points now show the CO abundance ratio for the corresponding temperature and gas density at each point in the disk. [Adapted from Diop et al. 2024]
The Top Contributors
After running their model and machine-learning algorithms, the authors found that the log of gas density is the most important factor in determining CO abundance. They note that this makes sense because the abundance of CO is typically related to the abundance of hydrogen (H2) gas in a disk. Interestingly, they also find that the log of gas density squared decreases with increasing CO. This is because at higher densities there is a competing effect of depleting CO as it freezes into ice in dense, cold regions of the disk.
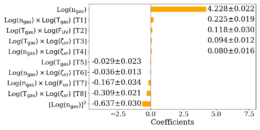
Figure 3: The coefficients indicating positive or negative correlation of the parameters listed on the left with CO abundance. Tgas and ngas correspond to the gas temperature and density, while FUV is the ultraviolet flux from the star, ζcr describes the cosmic ray rate, and ζxr is the X-ray rate. [Diop et al. 2024]
Know Thy Disk
This study provides a great proof of concept for the ways in which machine learning can be used to identify complex relationships in datasets — a particularly useful tool for studying the large number of entwined reactions involved in chemistry. It also brings up the important issue that molecular abundances can be influenced by many different factors, so we should consider disks as holistically as possible and always think about how multiple parameters may interact for a particular disk!
Original astrobite edited by Lucas Brown.
About the author, Isabella Trierweiler:
I’m a fifth-year grad student at UCLA. I’m interested in planet formation, and I study the compositions of exoplanets using polluted white dwarfs. In my free time I like knitting, playing train games, and growing various fruit trees.