Astronomers observe and model exploding stars to understand the details of stellar evolution, map the chemical enrichment of our galaxy, and even estimate the expansion of the universe. Today we take a look at five recent approaches to supernova science, from assessing the behavior of a single star to making plans for future surveys that will detect thousands of supernovae every year.
Radio Investigation of an Exploding Star
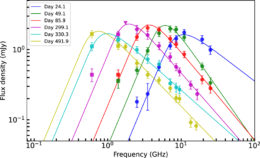
Radio spectra observed with the Jansky Very Large Array (circles) and the Giant Metrewave Radio Telescope (squares) compared to best-fitting models (solid lines). [Nayana A. J. et al. 2022]
While it’s possible to identify the star that went supernova in images taken before the explosion, researchers rely on models of supernova light curves to extract details about the material surrounding the star, which can hold clues about the star’s behavior before it went supernova. The team modeled SN 2016gkg’s radio light curves and spectra, simulating the effects of a shock wave plowing into material previously released by the star. The results suggested that the shock wave from SN 2016gkg expanded outward at 10% the speed of light into stellar wind material produced in the last hundred years of the star’s life.
Accounting for Cooling
C.-I. Björnsson (Stockholm University, Sweden) investigated the radio-wavelength behavior of another supernova, SN 2020oi. SN 2020oi is classified as a Type Ic supernova, which means the explosion resulted from the collapse of a massive star that had lost its hydrogen envelope. Type Ic supernovae are distinguished from other types of supernovae by their spectra, which have weak or no spectral features from silicon or helium.
Björnsson modeled the supernova’s radio emission, which is largely produced by electrons traveling in helical paths around magnetic field lines. Where Björnsson’s modeling differs from previous modeling efforts is the inclusion of radiative cooling by these spiraling electrons in the spectral fitting process, rather than assessing the cooling rate after the fitting is done. The author demonstrated that accounting for cooling during the fitting process alters the inferred properties of the event; for example, while previous analyses have invoked a varying pre-explosion mass-loss rate to explain some features of SN 2020oi’s light curve, Björnsson found that incorporating cooling removed the need for a changing mass-loss rate.
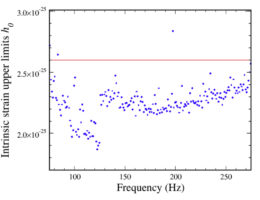
Upper limits on the intrinsic strain (a dimensionless quantity related to the amplitude of a gravitational wave; shown here in blue points) compared to an indirect limit on the strain set by the conservation of energy. [Adapted from Owen et al. 2022]
A Search for Gravitational Waves
SN1987A is one of the best-studied supernovae, but astronomers are still debating what it left behind. Multiple lines of evidence suggest that the explosion resulted in a neutron star — a city-sized stellar core so compressed that protons and electrons are squished together into neutrons — but the remnant has never been detected directly. Recently, a team led by Benjamin Owen (Texas Tech University) searched for gravitational waves from the purported neutron star. Unlike two objects colliding, which produces a sudden gravitational wave chirp, a young neutron star would emit gravitational waves constantly, powered by the slow spin-down of the rapidly spinning star.
Guided by knowledge of the neutron star’s likely distance, age, and size, the team searched through observations from the Laser Interferometer Gravitational-Wave Observatory (LIGO) but found no signal. However, the search allowed them to place upper limits on the neutron star’s gravitational wave emission and place constraints on the object’s properties, such as its ellipticity and magnetic field strength. As more precise search methods and gravitational wave data become available, Owen and collaborators anticipate more exacting searches will be possible in the future.
Preparing for the Future
Brian Hsu (Center for Astrophysics ∣ Harvard & Smithsonian) and collaborators looked ahead to future surveys, like the Vera C. Rubin Observatory Legacy Survey of Space and Time (LSST), which will produce a vast archive of photometric data. While LSST presents an incredible opportunity to study transient events, including supernovae, it also presents a challenge — how do we use photometric data to identify transient events that normally require spectroscopic data to classify? As an example, the authors considered superluminous core-collapse supernovae, which account for just 0.1% of all core-collapse supernovae but are overrepresented in surveys due to their extreme brightness (10–100 times brighter than a typical supernova).
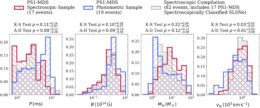
Posterior distributions of magnetar parameters derived from light curve modeling. Click to enlarge. [Hsu et al. 2022]
Studying Standard (and Over-Luminous) Candles
Not all supernovae are the result of massive stars collapsing; Type Ia supernovae occur when a stellar companion contributes mass to a white dwarf — an Earth-sized stellar core left behind when a low- or intermediate-mass (<8 solar masses) star exhausts its supply of hydrogen and loses its outer layers. All Type Ia supernovae are hypothesized to occur when a white dwarf reaches 1.44 solar masses, resulting in explosions of (theoretically) identical brightness. This makes Type Ia supernovae extremely valuable as standard candles, helping researchers gauge the distances to galaxies and even study the expansion of the universe.
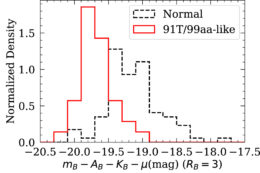
Histogram of absolute magnitude during peak brightness of typical Type Ia supernovae (black) and over-luminous “91T/99aa-like” Type Ia supernovae (red). [Adapted from Yang et al. 2022]
Because the two types of supernovae fade similarly in the months after exploding, Yang and collaborators point out that identifying over-luminous supernovae as they approach peak brightness is necessary. Otherwise, these over-luminous supernovae might be mistaken for typical supernovae, biasing calculations of cosmological distances. Accounting for differences in the light curves of Type Ia supernovae will become especially important for interpreting the results of future all-sky surveys, since these surveys will discover an outsize number of over-luminous Type Ia supernovae.
Citation
“Radio Evolution of a Type IIb Supernova SN 2016gkg,” Nayana A. J. et al 2022 ApJ 934 186. doi:10.3847/1538-4357/ac7c1e
“Radio Spectra of SN 2020oi: Effects of Radiative Cooling on the Deduced Source Properties,” C.-I. Björnsson 2022 ApJ 936 98. doi:10.3847/1538-4357/ac87aa
“First Constraining Upper Limits on Gravitational-wave Emission from NS 1987A in SNR 1987A,” Benjamin J. Owen et al 2022 ApJL 935 L7. doi:10.3847/2041-8213/ac84dc
“Photometrically Classified Superluminous Supernovae from the Pan-STARRS1 Medium Deep Survey: A Case Study for Science with Machine-learning-based Classification,” Brian Hsu et al 2022 ApJ 937 13. doi:10.3847/1538-4357/ac87ff
“Using 1991T/1999aa-like Type Ia Supernovae as Standardizable Candles,” Jiawen Yang et al 2022 ApJ 938 83. doi:10.3847/1538-4357/ac8c97