Geomagnetic storms — disturbances in Earth’s protective magnetic shield caused by oncoming solar particles — can have real-world consequences. A recent research article explores how machine learning can be used to create an early warning system for these events.
Geomagnetic Storms on the Horizon
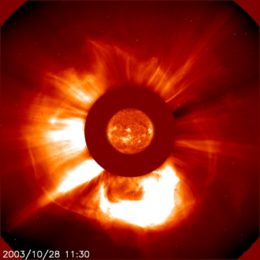
A white-light image of a coronal mass ejection taken by the Large Angle and Spectrometric Coronagraph. An extreme-ultraviolet image of the Sun is placed at the Sun’s location. [SOHO/LASCO, SOHO/EIT (ESA & NASA)]
In a recent publication, a team led by Andreea-Clara Pricopi (Technical University of Cluj-Napoca, Romania) tested the ability of machine learning to predict whether a coronal mass ejection will disrupt Earth’s magnetic shield. This technique may provide a way to anticipate geomagnetic storms days in advance.
An Expansive Sample
Machine learning is a relatively new technique in which computers are trained on a set of inputs with known outcomes. The trained computer can then predict the outcomes of a fresh set of inputs.
Pricopi and collaborators took as inputs the speed, angle, and acceleration of coronal mass ejections identified in white-light images, as well as a measure of the overall solar flare activity. The corresponding output is a measure of how disrupted Earth’s magnetic field became, known as the disturbance storm time index. The team trained the model on these inputs and outputs for a subset of 24,403 coronal mass ejections observed between 1996 and 2014, 172 (0.7%) of which caused geomagnetic storms.
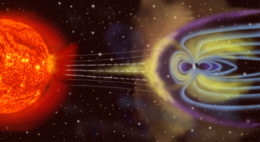
Artist’s impression of solar particles interacting with Earth’s protective magnetic shield, or magnetosphere, causing a geomagnetic storm. [NASA]
Prioritizing Powerful Events
Pricopi and coauthors trained their models on 80% of the data set, reserving the remaining 20% for testing the models’ performance. In order to push the models to prioritize finding geomagnetic storms, the team tested several strategies, including penalizing models that misclassified these events and creating synthetic storms based on real data to bulk up the sample size.
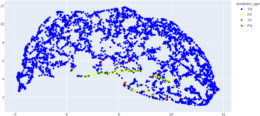
A visualization of the best model’s performance on the 20% of the data set reserved for testing. The color of the symbols indicates whether the model result was a true negative (TN, blue), false positive (FP, yellow), true positive (TP, green), or false negative (FN, red). Click to enlarge. [Pricopi et al. 2022]
These results show that machine learning can be used to predict geomagnetic storms days in advance using a limited number of inputs. However, the authors acknowledge that models that incorporate data from later in a coronal mass ejection’s evolution are more accurate. This suggests that the technique described in this work could be used to flag potentially damaging events, passing them to more precise models to get more information and improve our ability to prepare for an oncoming storm.
Citation
“Predicting the Geoeffectiveness of CMEs Using Machine Learning,” Andreea-Clara Pricopi et al 2022 ApJ 934 176. doi:10.3847/1538-4357/ac7962