Star catalogs are critical to astronomy research. However, they’re only as reliable as the methods used to create them. As telescopes probe further and fainter regions of the sky, how can we ensure that our methods of catalog creation extract as much information as possible from the returned images?
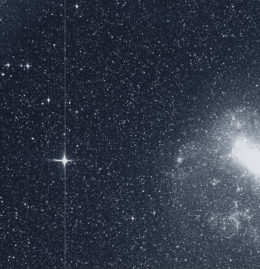
The “first light” image from the Transiting Exoplanet Survey Satellite. This is a good example of an astronomical research image, featuring the distortion of star shapes and crowded regions. The crosses typically accompany bright stars and are caused by the instrument doing the imaging. The object on the right is the Large Magellanic Cloud and the bright star on the left is R Doradus. [NASA/MIT/TESS]
To Get to the Point
The start of any star catalog is an image taken by a telescope. These images typically look like black and white photographs of the night sky, and the wavelengths of light used to make them are set by a filter, also known as a band. To make a catalog, you just need to identify stars in an image.
This isn’t as straightforward as it sounds, though! Stars, which would ideally appear as points, look like distorted circles due to atmospheric effects and electronics. Brighter ones can drown out fainter ones. They can also overlap, making it hard to tell them apart in crowded regions like the hearts of galaxies. And as telescopes get better, the images they produce will feature fainter stars and consequently be more crowded.
To efficiently create catalogs in the future, we need improvements on our methods of star finding. In a recent study, Richard Feder (California Institute of Technology) and collaborators used a technique called probabilistic cataloging (PCAT) to identify stars in the globular cluster Messier 2 (M2) and compared their results to existing catalogs on M2.
Assuming We Can Have More Than One Catalog
A catalog is generated by applying assumptions of what a star should look like and how bright it should be. This brightness threshold is a key assumption to catalog creation — too low and false stars may be let in, too high and real stars may be left out.
Traditional methods of catalog creation are one and done; assumptions are made and a single catalog is generated per image. In PCAT, multiple catalogs are generated per image, each with a different set of assumptions. This allows PCAT to have catalogs with very low brightness thresholds and identify sources (star candidates) that, while not bright enough to be confidently identified as stars, can influence the sources around them. The multiple catalogs are eventually collapsed into a single one after source influences and other factors have been accounted for.
A Few Levels Deeper
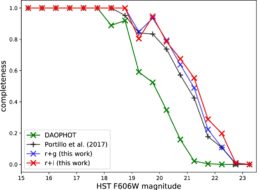
The completeness of different catalogs obtained from the SDSS images relative to the Hubble images. The x-axis is stellar brightness in Hubble magnitudes and the y-axis is completeness, with 1.0 meaning that all the Hubble stars were recovered in the SDSS data and 0.0 meaning no Hubble stars were recovered. DAOPHOT corresponds to the traditional catalog, Portillo et al. corresponds to PCAT applied to a single band, and r+g and r+i refer to the combinations of multiband images used in this work. [Adapted from Feder et al. 2020]
M2 has the advantage of having existing catalogs generated from images taken by the Sloan Digital Sky Survey (SDSS) and the Hubble Space Telescope (HST). Feder and collaborators worked with SDSS images taken with the g, r, and i bands, using the HST catalog as a “complete” catalog to determine how well they were finding stars. They also compared their results against a traditional catalog generated from SDSS images.
Feder and collaborators found that they recovered stars ~0.4 magnitude fainter when using the multiband images instead of the single band ones. They went 1.5 magnitudes fainter than the traditional SDSS catalog and could confidently recover stars brighter than 20th magnitude. They also developed a more reliable method of determining star positions accurately across images.
With a better understanding of what assumptions can be made for images taken by different telescopes, PCAT could be widely applied to astronomical data in the near future. Stay tuned!
Citation
“Multiband Probabilistic Cataloging: A Joint Fitting Approach to Point-source Detection and Deblending,” Richard M. Feder et al 2020 AJ 159 163. doi:10.3847/1538-3881/ab74cf
1 Comment
Pingback: From AAS NOVA: “A Stellar Method of Catalog Creation” | sciencesprings